Here’s what’s holding back your generative AI project
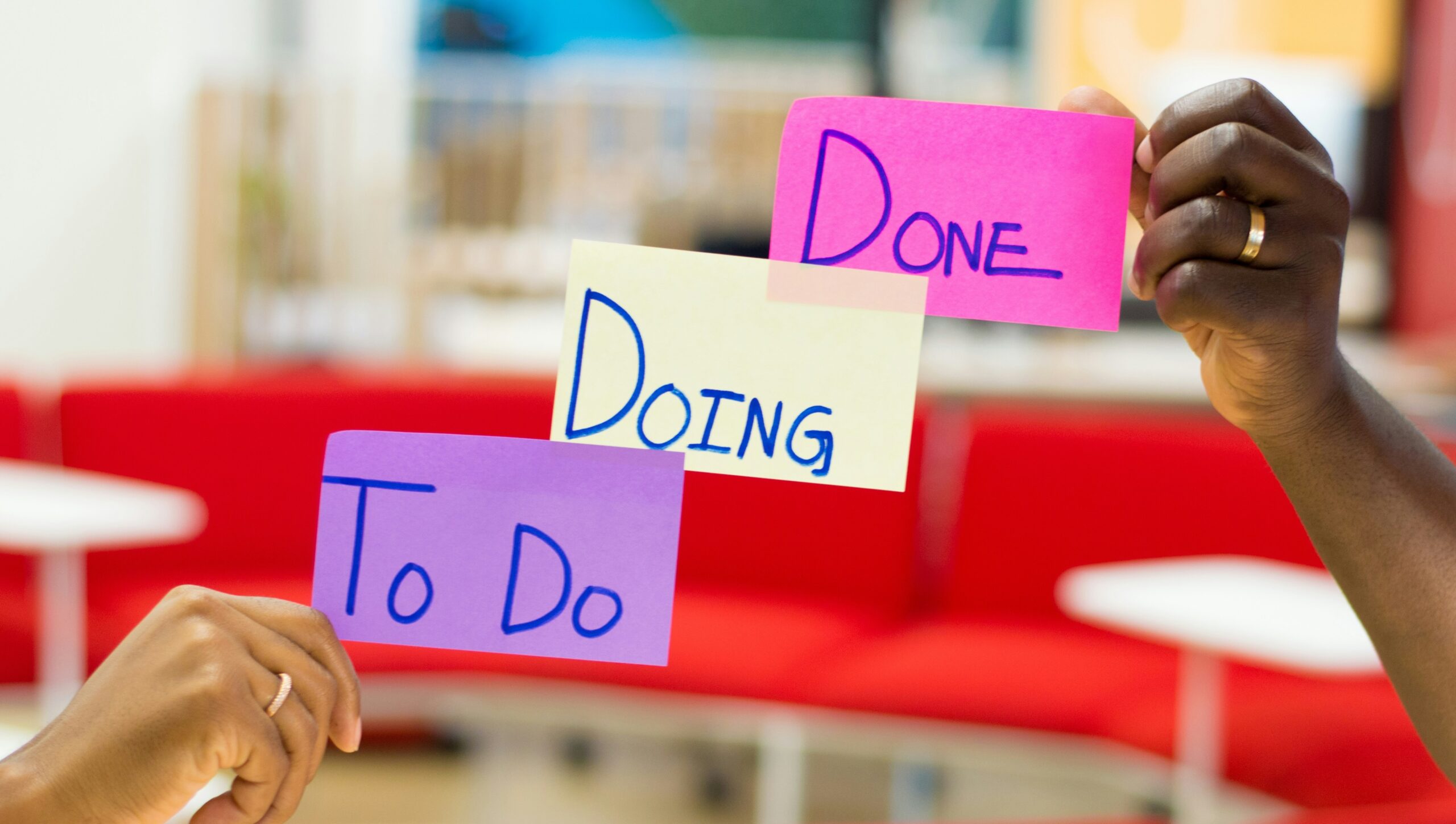
It's been just over two years since OpenAI shocked the world with the release of ChatGPT. It has had a profound impact on just about every organization, as executives try to figure out how to best put generative AI to work inside their companies. Yet multiple studies suggest that firms are still having a hard time getting beyond experiments and proofs of concept to scalable applications with a measurable impact on productivity and revenue.
By now it’s clear that C-suite executives recognize the vast potential of generative AI to increase efficiency in every aspect of their business. In fact, most companies are at some stage of implementing AI projects with companies looking for the right balance between building versus buying and all that goes into that.
But companies are struggling to scale these experiments. Deloitte reported that “over two-thirds of respondents [to a recent survey] said that 30% or fewer of their current experiments will be fully scaled in the next three to six months.”
Regardless of how your company may be implementing your generative AI applications, three recent studies – the one from Deloitte, one from Boston Consulting Group and one from Kearney and Futurum – found that there are a number of factors that are holding companies back when it comes to scaling generative AI solutions, which can be divided into two areas: people and technology.
The people problem
People are always going to be a significant factor when it comes to successfully implementing generative AI projects. Humans are complex and emotional and that makes it even more challenging to build this kind of technology. The people problem actually falls into several categories.
For starters, as BCG found, there is a huge cultural component. Making a shift this substantial involves a massive change management effort, and it’s a big part of what’s holding back large organizations as they try to scale generative AI projects. “Winning with AI is a sociological challenge as much as a technological one. The soft stuff — reimagining workflows, upskilling talent, and driving organizational change — turns out to be the hard stuff.”
The Kearney-Furturum report saw the people problem through a job security lens. It’s hard to get people to buy in if they think they are training the technology to take their jobs. Managers have to do a better job of communicating the value of this technology for the workers, as well as the organization, to get buy-in. Ultimately, as one consultant told me, most people are looking out for their own self interest, and if they think AI is coming for them, they aren’t going to cooperate.
“By proactively addressing job-security fears and skill gaps, CEOs can uphold near-term performance while preparing employees for future AI use cases. Failing to do so often triggers cultural friction, stalled pilots and missed opportunities,” the report found.
Another issue, according to Deloitte, is the lack of people with AI skills. It’s important for companies to train employees in the skills they need for the future, which in turn should contribute to more successful projects and more buy-in as employees begin to see the value of these tools. “Without adequate workforce buy-in and training, even the most powerful GenAI solutions can fail to deliver the expected outcomes,” Deloitte found.
The tech problem
While the people problem is hard enough to solve, the tech problem is even more daunting. No company can escape their existing tech stack. Most large companies have some level of technical debt, which involves structural problems in tech systems that have built up over time, and can make it difficult to implement more modern software solutions like generative AI.
As Box CEO Aaron Levie pointed out, while many companies have started on their journey to become an AI enterprise, there are many obstacles and challenges to get there. “What's remarkable about AI is I think basically almost every enterprise fully understands that they have to become an AI-first company and adopt AI. Yet at the same time we’re very early in the AI journey” Levie told FastForward. He said companies need to consider what their AI system is going to look like in terms of structure, technology, models and so forth.
As Levie stated, a major obstacle to AI success is the data problem. If your data isn’t in order, it’s going to make it challenging to use it to train models. In fact, the Kearny-Futurum report found that data readiness was a primary factor holding companies back from successfully implementing generative AI projects. “Nearly two-thirds of CEOs cite disconnected or low-quality data as the main barrier preventing AI solutions from scaling beyond pilot phases, underscoring how critical robust data readiness is to any AI initiative.”
Deloitte reported that older systems, an inevitability in most large organizations, are also holding companies back. “Yet interviews reveal that legacy infrastructures in these organizations often come with deeply siloed data and complex governance hurdles, slowing down early pilots despite their commitment to robust, long-range returns.”
Another impediment to success is lack of a modern governance structure designed specifically for the AI era. According to Kearney-Futurum, just 22% of organizations have set up AI governance councils to consistently track bias-detection metrics. “While many firms rely on existing committees or compliance teams, formal governance frameworks — complete with cross-functional councils — prove more adept at curbing risks like bias, regulatory pitfalls and ethical missteps,” according to the report.
BCG found that beyond tech, other issues involve lack of strategic focus and aiming too low with project goals. “Most companies aim too low, prioritizing smaller-scale, productivity-focused initiatives. Leading companies allocate more than 80% of their AI investments to reshaping key functions and inventing new offerings,” the report concluded. Companies need to look at what successful companies are doing and emulate that if they hope to achieve similar results.
As companies struggle to scale their generative AI initiatives, it’s worth understanding why, and reading reports like the ones cited here can be a good starting point. Keep in mind that this isn’t easy and many of the problems you face are common to all large organizations. It’s going to require strong and focused leadership, even more than making the right technology decisions, to overcome these obstacles to success.