IBM sees an AI future with smaller, more focused models in the enterprise
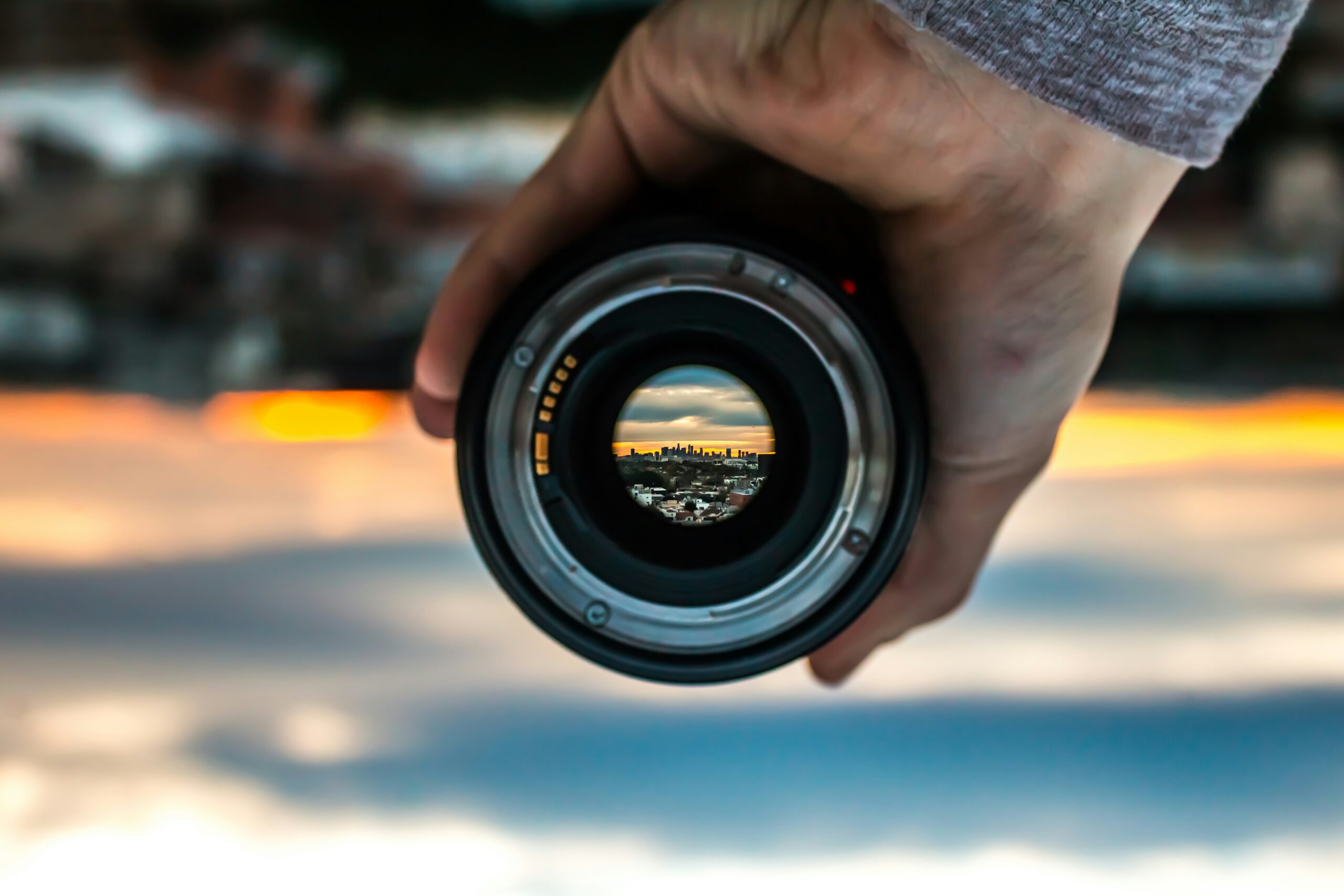
While there was lots of news coming out of IBM Think 2025 last week in Boston, one thing that stuck out for me was the emphasis on smaller models. Bigger isn’t necessarily better, especially in the enterprise, where companies need to concentrate on their own data to get an AI advantage.
In a training article from February Splunk’s Muhammad Raza explained the key differences between small language models and large language models. “Small language models (SLMs) have fewer parameters and are fine-tuned on a subset of data for a specific use case. Large language models (LLMs) are trained on large-scale datasets and usually require large-scale cloud resources,” he wrote.
When I spoke to David Cox, the VP for AI models at IBM Research in March, he said that being the biggest shouldn’t necessarily be the goal, nor does trumpeting benchmarks serve customer needs. “I think now any model that comes out, either you have to go really, really deep and shocking on one capability, but often at the sort of detriment of other capabilities, or you have to be the biggest, baddest benchmark-busting model,” Cox told FastForward.

While Cox’s statement might be somewhat self-serving, there is some truth to it. It was a point I made instinctively early on in the generative AI revolution in an April 2023 TechCrunch article: “There is some thinking that in order to work in the enterprise, the models will have to be flexible enough to deal with proprietary company data for model training, and if that’s the case, the future could involve smaller and more focused models,” I wrote at the time.
Two years later, IBM CEO Arvind Krishna was trumpeting the same idea at last week’s keynote. “If you think about the massive general purpose models, those are very useful, but they are not going to help you unlock the value from all of the data inside the enterprise,” he said. “To win, you are going to need to build special purpose models, that are much smaller, tailored for a particular use case, and that can ingest the enterprise data and then work,” he said.
When I wrote that article back in 2023, people suggested that, much like trying to put Google Search to work inside large enterprises, there wasn’t enough data to produce accurate or pinpointed results. Just as Google was good because it had the whole web, the big LLMs are good because they have access to massive sets of training data.
Enterprise cost considerations
This isn’t just IBM saying this though. In March, I moderated a panel on infrastructure at Human X in March, which included John Yue, co-founder and CEO at Inference.ai, who believes that looking beyond the mega models serves the majority of enterprise customers much better, especially as it relates to cost.
“We don't want to be deploying big models all the time just for simple tasks,” he said. “So I think we're going to start to see a proliferation of smaller models.” Cost is a big factor. As Cox pointed out, smaller models need fewer GPUs and that’s going to appeal to large companies with ballooning AI bills, enabling them to experiment in ways not possible with tighter cost constraints.
“If you can get a smaller corpus of data to train the model with, you will need a smaller number of GPUs to go out and run the model, and that then opens up avenues in very different ways than otherwise would have not been opened,” he said.
We don't want to be deploying big models all the time just for simple tasks. So I think we're going to start to see a proliferation of smaller models.
FluidStack CEO César Maklary, speaking on the same Human X infrastructure panel, sees a world in which a few companies do the training and most companies rely on models for inference and application building. “I'm seeing maybe 20 companies who can again afford those massive training runs from scratch, and who have still the financial capabilities to do so, and then to be able to foot those R&D expenses, and then really the 99% of demand coming from inference and fine tuning, especially inside enterprises who really try to unlock the value of those next generation models,” he said.
Can bigger be better?
It’s worth noting, however, that not everyone sees SLMs as the only answer. For example, Microsoft’s VP of AI Luis Vargas sees a mixed approach as better. “Some customers may only need small models, some will need big models and many are going to want to combine both in a variety of ways,” Vargas said, ironically in a blog post on the release of Microsoft’s Phi-3 small language models last year.
There are arguments to be made that larger models with access to more information can just naturally produce more accurate results. It’s a position that full stack AI vendor SambaNova took in a blog post last year: “The larger the AI model, the more accurate, meaningful, and functional the results that it provides will be, so running the largest models is critical. The challenge then becomes choosing the right model and platform to power continuously expanding, very large models,”
Regardless, as AI continues to evolve, IBM believes that for the majority of enterprises, success will come not from bigger models, but from more focused ones. While it's a position that makes sense for many companies, it’s worth remembering that not everyone agrees, and enterprise customers will need to find AI solutions that work best for their particular needs.
Featured photo by Devin Avery on Unsplash