The AI project failure paradox
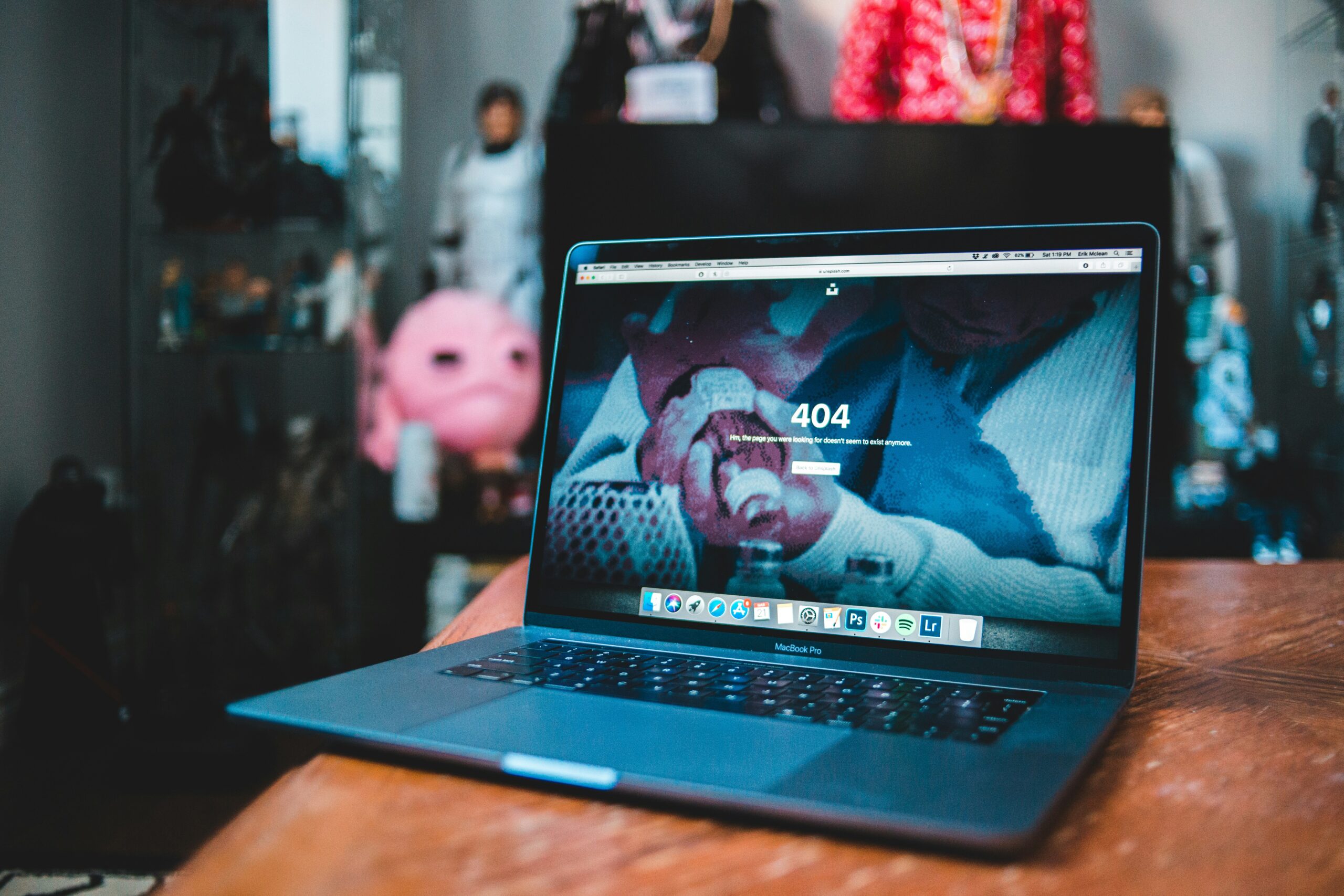
Yet another report emerged this week, confirming that large companies continue to struggle to implement successful AI projects. This time it was Accenture, and it told the same story we heard in reports at the end of 2024. Only about a third of AI proofs of concept and experiments are making it into production.
Even worse, Accenture found that just 13% of companies had reported seeing any kind of enterprise value from these projects. Those are dismal numbers, and yet they are in line with similar research conducted by BCG, Deloitte and Futurum at the end of last year.
At this point, we know there is a huge vendor push to sell generative and agentic AI products and services. We know that there is a massive ongoing data center building program, aimed at anticipating the growing demand for resources required to run AI workloads. Yet customers seem to be lagging behind all of this activity, at least according to all this research. This is coming from four different reports from reputable firms, all finding similar results. It's hard to ignore.
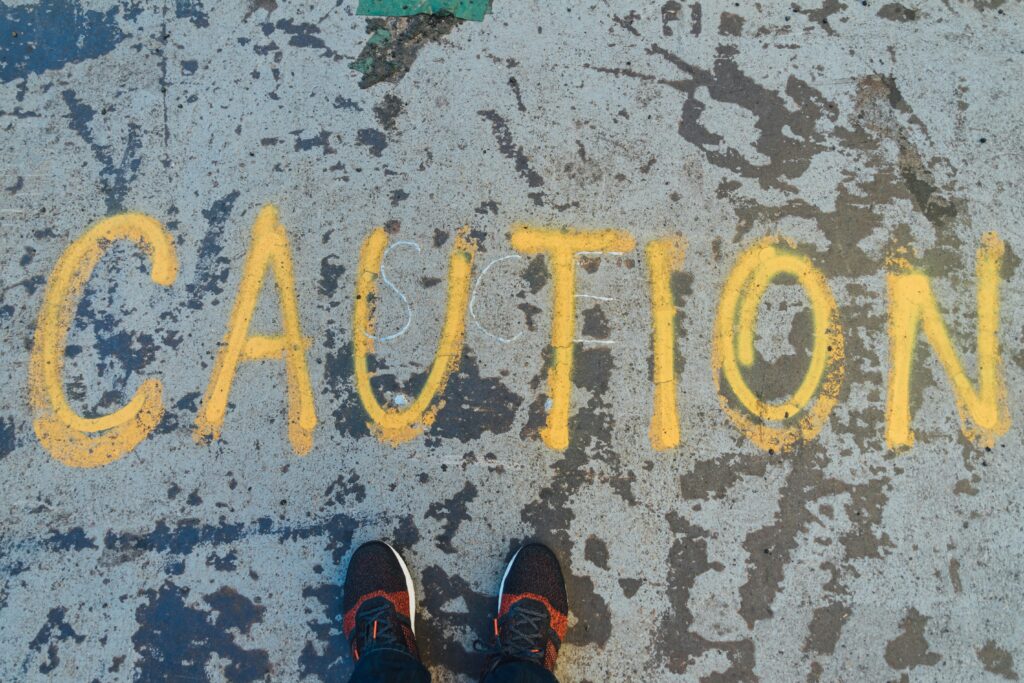
Photo by Goh Rhy Yan on Unsplash
Just this week on LinkedIn, Box CEO Aaron Levie, who writes frequently about AI in the enterprise, was observing that AI had the advantage of executive backing that other technologies like cloud computing historically have lacked. “Up and down the executive chain in most companies, just a couple of years in, and it’s clear that every enterprise knows they will have an AI strategy,” Levie wrote.
The strong executive backing combined with an attempt to build coherent AI strategies makes these consistently low success rates even more baffling. Lack of executive leadership is often cited as a major factor when it comes to project failure inside large companies. Of course, it could also be attributed to some percentage of rank and file employees feeling threatened by this technology, and without full organizational buy-in projects can falter.
But perhaps what we’re seeing is actually a natural and expected lag in production. In a recent interview with economist Paul Krugman, Stanford Professor of Economics Erik Brynjolfsson suggested that the current AI production gap may be understandable and something that happens with every major technological shift — a pattern he calls the productivity J curve.
“What happens is, at first you have to reskill your workforce, put a lot of time into training, and people on their own have to figure things out. You build new business processes, even new products and services, new organizations,” he said. This should sound familiar, as many large companies (along with many of us as individuals) are experiencing the same challenges with AI today.
"What happens is, at first you have to reskill your workforce, put a lot of time into training, and people on their own have to figure things out."
As Brynjolfsson pointed out, we saw a similar dynamic at work in the 19th century with the advent of electricity. At first companies simply replaced their steam engines with electric motors, and for a while didn’t see much of a productivity gain. But over time as they figured out how to best implement the new technology, they started installing smaller motors across the factory, leading to entirely new concepts like the assembly line, which resulted in an explosion of productivity.
Today, we're still swapping old tech for new, when we truly need to reimagine how we work. Until that happens, I suspect productivity gains will remain elusive, and AI project success rates will continue to lag. Yet if history is any guide, smart managers will eventually discover their ‘assembly line moment,’ and progress should accelerate. Until then, I’m guessing we will continue to see reports with disappointing results.
~ Ron
Featured image by Erik Mclean on Unsplash