With a strong data foundation, Capital One was more prepared for generative AI than most
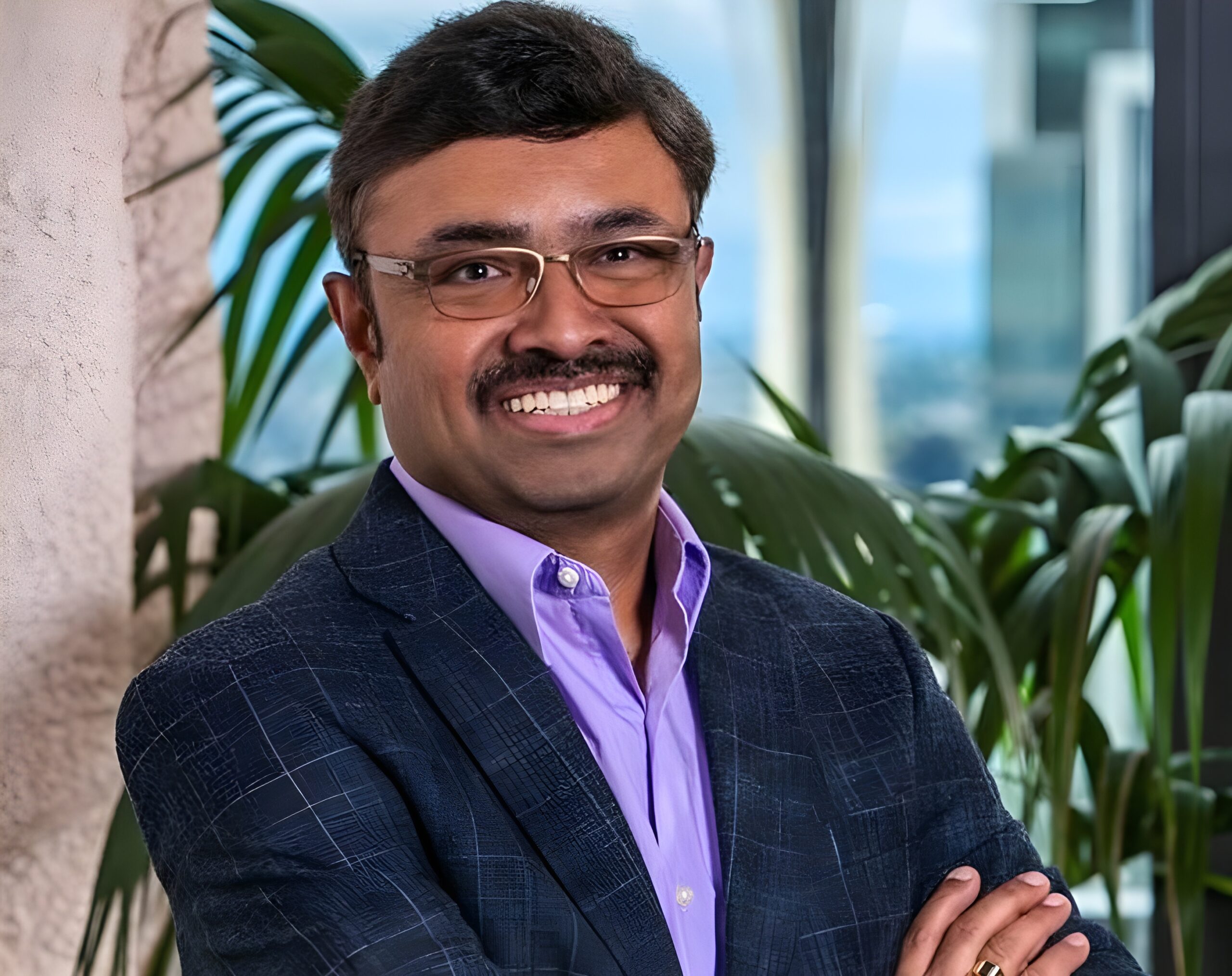
Capital One launched 30 years ago with what was an unusual approach at the time. Its goal was to harness data to gain deeper insights into its customers. Two decades later in 2012, the company began its cloud transformation journey at a time when many large organizations were holding back.
That modern technology approach laid the foundation for the Capital One to make a fairly smooth transition to generative AI when it came along. Prem Natarajan, chief scientist and head of enterprise data and AI, joined the financial services giant just two years ago, but is building on decades of progress that is enabling his firm to build AI applications, rather than buying off-the-shelf solutions.
“Culturally, there is a deep reverence for data in the company’s DNA,” Natarajan told FastForward last week at the Human X conference in Las Vegas. “And that manifests itself in many ways such as how much data is used for decision making and how insights are organized around tools and techniques that help us get insights from data.”
Today, a deep relationship with AWS, combined with a modern data stack in the cloud, allows them to move very quickly. Contrast that with a company that has to get the data in order first, which is the case with a lot of organizations, and it gives Capital One a big advantage.
To the cloud
As the company made its transition to the cloud, it continued to be a data-first organization. That led to exploring machine learning as it developed over the last decade to build more advanced applications. It was yet another decision that set them up for the more recent developments around large language models and generative AI as they have come to the fore.
Natarajan sees how building upon these two inflection points, data and cloud, was so important in contributing to their base of knowledge. “We learned to address a lot of the challenges around how you consume data to build these models and test these models,” he said.
“When we wanted to run our first limited experiments, let's say with transformers and genetic techniques, we were not assembling all of the data for the first time because we had been using the data in these earlier models."
Build first mentality
Today, Capital One is well situated to build applications on top of large language models. While no large organization is 100% build or 100% buy when it comes to applications, Natarajan says companies tend to lean one way or the other. “I think we are instinctively more on the build side, but having said that, we don't build everything.” So the question is what makes them lean one way or the other.
He mentions a couple of key indicators. For starters, if the application relies on proprietary Capital One data, they lean towards building. “We will build when we think we are going to embed some business insights or understanding of the business into this technology, in which case it is something that's kind of fundamentally proprietary to us,” he said.
The only way for my data advantage to become my AI advantage is if I can customize the models with my data, and I cannot customize closed source models.
Being a financial services company, they have to take the regulatory component into account, and what he refers to as a black box problem. Regulators abhor a black box because it’s hard to know how it works, and whether or not a company is protecting the data and complying with the rules around it. So in cases where having a vendor’s black box versus having your own (where you know what’s going on inside), they will lean towards proprietary to placate the regulators. He says this is known as 'the black box hierarchy problem.'
When those factors aren’t in play, and it’s not something fundamental to the core business, then they will buy, he says.
Looking to open source
When his group decides to build, it invariably involves open source tools. “We are really anchored on open source, so we like Llama (Meta’s open source large language model) for that reason,” Natarajan said. That’s because open source is flexible enough to really take advantage of all the data they are collecting.
“The only way for my data advantage to become my AI advantage is if I can customize the models with my data, and I cannot customize closed source models." He says that when they talk about open source at Capital One, they really mean open weights, the ability to see the numerical value assigned to each individual behavior in the model, rather than seeing the data that was used, or the way the model was trained.
He says that even though open source/weight models are often a couple of months behind the state of the art, given the fact he can see those weights, the lag is a trade-off he’s willing to make because he knows the open source models will catch up with best models in fairly short order.
Natarajan says this blend of culture and technology has helped enormously over the years as the company has moved through the different phases of computing to get to today. Certainly having a data-centric approach has prepared the company to take advantage of the current surge brought on by large language models and generative AI.
Feature photo courtesy of Capital One.